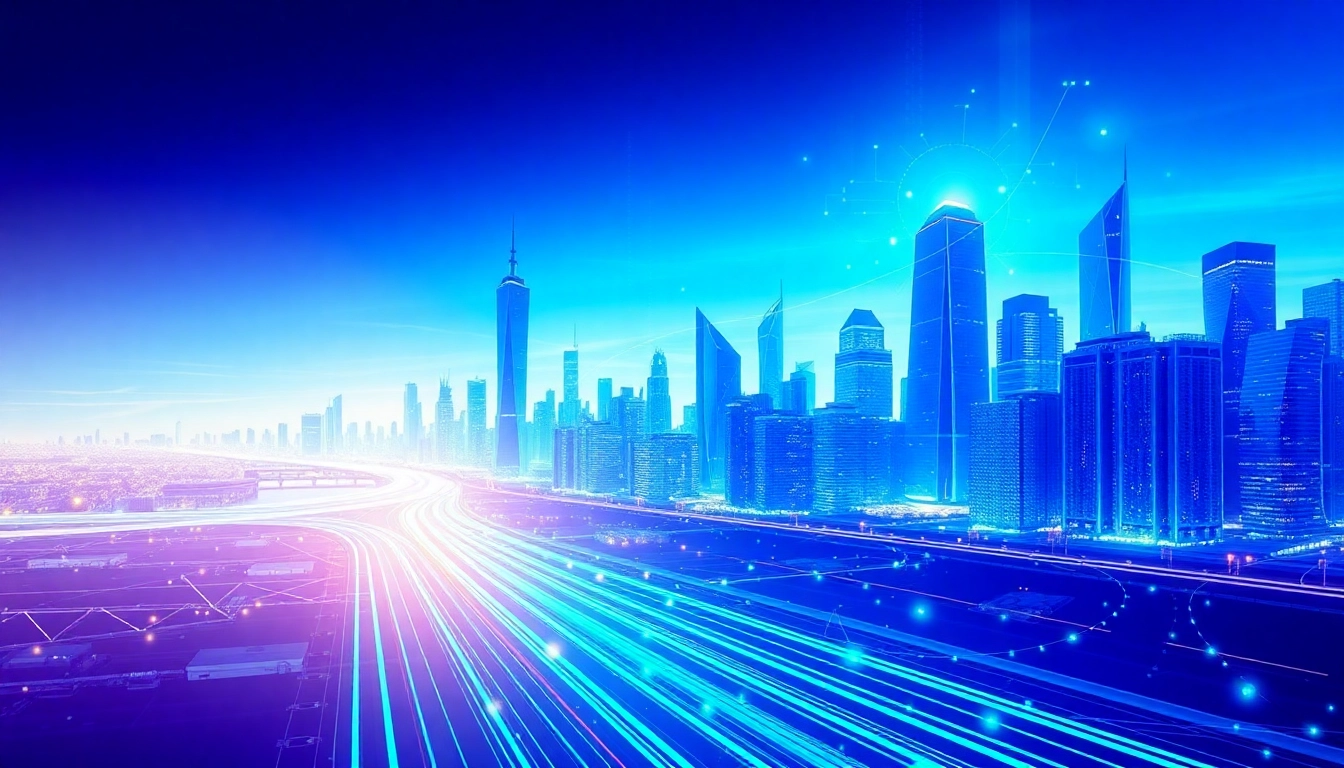
What is Agentic AI?
Definition and Overview
Agentic AI represents a significant shift in the landscape of artificial intelligence, defined by its capacity for autonomy and self-determination in complex tasks. Unlike traditional AI models, which primarily rely on specific inputs to generate outputs, Agentic AI systems can independently assess their environment, identify objectives, and take actions without constant human oversight. These capabilities hinge on advanced algorithms that combine machine learning, data analytics, and decision-making processes, enabling their use in sectors ranging from finance to healthcare and beyond.
Key Features of Agentic AI
Agentic AI is characterized by several key features that distinguish it from conventional AI models:
- Autonomy: Agentic AI operates without the need for continuous human intervention, making independent decisions based on real-time data.
- Learning and Adaptation: These systems leverage machine learning techniques to adapt their strategies and improve their effectiveness as they encounter new information.
- Complex Problem Solving: Capable of tackling multi-step problems, Agentic AI employs sophisticated reasoning and planning mechanisms to achieve complex objectives.
- Planning and Execution: Beyond merely responding to inputs, Agentic AI can devise strategies, prioritize tasks, and execute actions to reach defined goals.
Comparison to Traditional AI Models
To fully appreciate the significance of Agentic AI, it’s essential to understand how it differs from traditional AI frameworks:
- Dependent vs. Independent Operation: Traditional AI typically requires human-facilitated inputs or guidance in decision-making processes, while Agentic AI operates autonomously.
- Static vs. Dynamic Learning: Conventional AI is often built around predefined datasets with limited learning capabilities, whereas Agentic AI continuously learns and adapts its understanding as new data becomes available.
- Execution of Tasks: Traditional AI may merely generate outputs based on input data (like text generation), while Agentic AI interacts with its environment to perform actions that impact real-world outcomes.
How Agentic AI Operates
Learning and Adaptation
At the core of Agentic AI’s functionality is its ability to learn from experiences and adapt to new circumstances. This is achieved through algorithms that incorporate machine learning techniques, allowing Agentic AI systems to process vast amounts of information and identify patterns over time. They utilize supervised, unsupervised, or reinforcement learning methods, depending on the context and goals. For instance:
- Supervised Learning: This technique is useful when historical data is available and can help the AI recognize outcomes based on labeled examples.
- Unsupervised Learning: In scenarios where no labeled data exists, Agentic AI can identify hidden patterns and clusters in input data.
- Reinforcement Learning: This paradigm allows the AI to learn by trial and error, receiving rewards or penalties based on the effectiveness of its actions.
Problem-Solving Capabilities
Agentic AI is designed to tackle complex challenges by utilizing its sophisticated planning and decision-making capabilities. By breaking down intricate problems into manageable parts, these systems can prioritize actions based on the anticipated outcomes and resource availability.
A practical example of this can be seen in supply chain management, where Agentic AI can optimize logistics by calculating the most efficient routes and allocating resources dynamically as conditions change, such as traffic patterns or demand fluctuations.
Case Studies in Action
Real-world case studies showcase the prowess of Agentic AI in various scenarios:
- Healthcare: In the medical field, Agentic AI systems analyze patient data to recommend treatments, predict disease outbreaks, or optimize resource allocation during critical situations.
- Finance: Financial institutions leverage Agentic AI to automate risk assessments, detect fraudulent transactions in real-time, and manage investment strategies autonomously.
- Telecommunications: Companies in this sector utilize Agentic AI to manage network traffic intelligently, enhancing service quality and reducing downtime through predictive maintenance algorithms.
Applications of Agentic AI
Industries Leveraging Agentic AI
Agentic AI’s applicability spans multiple industries, demonstrating versatility and efficacy:
- Manufacturing: In smart factories, Agentic AI helps streamline production processes, monitor equipment health, and manage supply chains.
- Transportation: Autonomous vehicles utilize Agentic AI for navigation and decision-making to ensure safe travel.
- Retail: E-commerce businesses are adopting Agentic AI for personalized customer experiences, inventory management, and demand forecasting.
Benefits of Implementing Agentic AI
The integration of Agentic AI within organizations offers a myriad of benefits, including:
- Increased Efficiency: By automating decision-making processes, businesses can drastically reduce operational costs and response times.
- Enhanced Precision: The ability to analyze real-time data ensures that decisions are made based on the most current information available.
- Scalability: Agentic AI can scale operations efficiently, adapting to increases in demand or modifications in operational needs without significant resource investments.
Real-World Examples and Outcomes
Real-world implementations of Agentic AI have demonstrated impressive outcomes:
- Walmart: The retail giant employs Agentic AI to optimize supply chain logistics, resulting in significant savings and improved customer satisfaction.
- GE Aviation: Using Agentic AI, GE Aviation enhances predictive maintenance on aircraft engines, minimizing downtime and enhancing safety.
- JP Morgan: The investment bank applies Agentic AI to automate document analysis and expedite financial reviews, improving operational efficiency.
Challenges and Limitations of Agentic AI
Ethical Considerations
While Agentic AI presents several advantages, it also raises ethical concerns that must be addressed:
- Transparency: The autonomous nature of these systems can obfuscate decision-making processes, making it difficult to understand how specific outcomes are reached.
- Bias: If the data fed into Agentic AI systems is biased, the output will likely reflect those biases, leading to unjust outcomes.
- Accountability: Determining responsibility for decisions made by autonomous systems can become complicated, especially in critical situations like healthcare.
Technical Limitations
Despite the potential of Agentic AI, there are notable technical challenges:
- Data Quality: Effective learning relies heavily on high-quality, relevant data. Incomplete or noisy data can significantly hinder performance.
- Understanding Context: While Agentic AI can analyze data, it may struggle with nuanced situations that require deeper contextual understanding.
- Integration with Existing Systems: Adopting Agentic AI often requires overhauling traditional processes, which can be resource-intensive and time-consuming.
Future Perspectives
The future of Agentic AI is promising, with continued research and development likely to address current limitations while expanding its capabilities:
- Advanced Learning Techniques: Ongoing advancements in machine learning may enable these systems to grasp context more effectively and improve accuracy in real-world applications.
- Human-AI Collaboration: Developing frameworks for collaboration between humans and Agentic AI could enhance efficiency and mitigate ethical concerns.
- Regulatory Frameworks: As adoption grows, the establishment of regulations governing the use of Agentic AI may become essential to ensure ethical standards are maintained.
The Future of Agentic AI
Emerging Trends
The landscape of Agentic AI is evolving rapidly, with several emerging trends that business leaders and tech enthusiasts alike should watch:
- Hybrid AI Systems: The integration of Agentic AI with generative AI models is expected to produce more versatile systems capable of creativity and innovation.
- Federated Learning: This approach helps create AI models that learn from decentralized data while improving privacy and security.
- Explainable AI: Growing demand for transparency in AI decision-making may lead to the development of mechanisms that clarify how Agentic AI reaches its conclusions.
Potential Innovations
Looking ahead, several innovative applications could further enhance Agentic AI functionalities:
- Smart Cities: Agentic AI can be integral in developing smart city solutions that intelligently manage resources and services.
- Cybersecurity: Leveraging Agentic AI in cybersecurity can enhance threat detection and response times, creating robust security measures.
- Quality Control: Manufacturing processes could see improvements through real-time monitoring and anomaly detection, leading to higher product quality and reduced waste.
How Businesses Can Prepare
As Agentic AI is expected to reshape many industries, here are several preparatory steps businesses can take:
- Invest in Data Infrastructure: Establishing a robust data collection and management framework will be crucial for effectively employing Agentic AI.
- Upskill Workforce: Training employees in AI literacy can foster a more competent workforce capable of adopting new technologies.
- Embrace Agile Methodologies: Being adaptable and responsive will enable organizations to pivot effectively as Agentic AI technologies evolve.