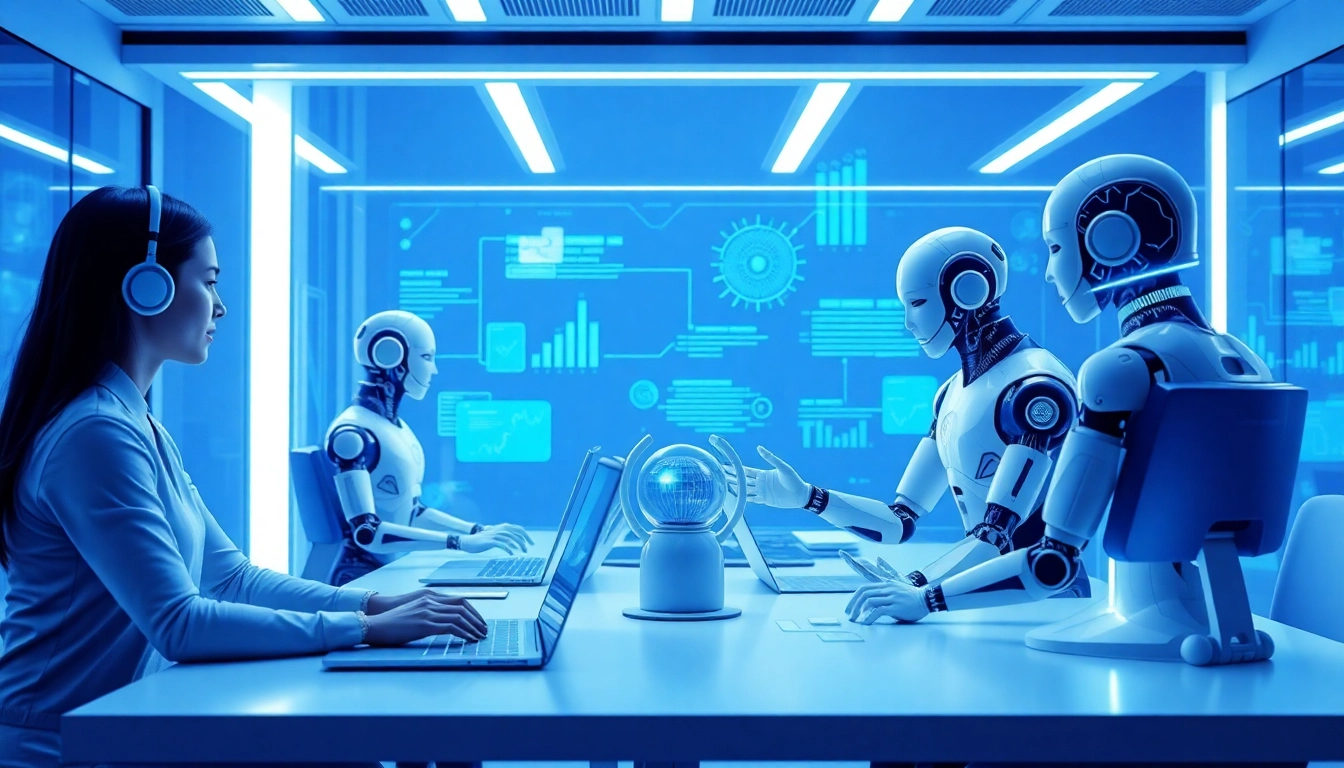
Introduction to Agentic Human AI
As technology continues to evolve at an unprecedented pace, the concept of Agentic Human AI has emerged as a groundbreaking frontier in the realm of artificial intelligence (AI). This paradigm encapsulates the ability of AI systems to operate autonomously, making decisions and taking actions aimed at achieving predefined goals without constant human oversight. With the advent of sophisticated machine learning models and algorithms, the need for effective human-AI interaction is more critical than ever.
Definition and Key Concepts
Agentic Human AI refers to AI technologies capable of performing tasks that traditionally required human intelligence, but now can execute them with a degree of autonomy. At its core, agentic AI blends the principles of autonomy, adaptability, and contextual understanding.
Some key concepts associated with agentic AI include:
- Autonomy: The ability of AI systems to operate independently in dynamically changing environments.
- Contextual Awareness: Understanding the nuances of the environment in which they operate, allowing for better decision-making and interactions.
- Goal Orientation: Acting towards achieving specific objectives without direct human intervention.
Historical Context and Evolution
The journey of AI began in the mid-20th century, but the emergence of agentic AI is relatively recent. Early computational algorithms were primarily rule-based, requiring explicit instructions to function. However, significant advancements in neural networks and deep learning have facilitated a shift towards more autonomous systems. The introduction of reinforcement learning models and probabilistic reasoning is pivotal in this transformation.
Major Players in the Field
Numerous companies and research institutions are at the forefront of developing agentic AI technologies. Key players include:
- IBM: Focusing on creating intelligent systems capable of autonomous operation across various sectors.
- Google: Innovating in machine learning applications that emphasize contextual decision-making.
- OpenAI: Pioneering advancements in AI that allow for complex interactions and autonomous problem-solving.
Core Components of Agentic Human AI
Autonomy and Decision-Making
Autonomy is a foundational component of agentic AI. Systems can analyze situations, weigh options, and make informed decisions without continuous human input. This capability is especially beneficial in environments characterized by improvisation and rapid change. For instance, in autonomous driving technologies, vehicles utilize sensor data and real-time processing to navigate, make decisions, and react to their surroundings safely.
Human-AI Collaboration Models
The synergy between humans and AI is crucial for maximizing the benefits of agentic systems. Various models exist, including:
- Complementary Collaboration: AI systems augment human capabilities by handling routine tasks, freeing individuals to focus on strategic initiatives.
- Cooperative Collaboration: In this model, AI systems and humans work together, with AI providing insights and analysis to support human decision-making.
Technologies Enabling Agentic AI
At the heart of agentic AI are several key technologies:
- Machine Learning: Algorithms that allow systems to learn from data and improve over time without being explicitly programmed.
- Natural Language Processing (NLP): Enabling machines to understand and respond to human language effectively.
- Computer Vision: The ability of AI systems to interpret and make decisions based on visual data, applicable in fields like surveillance and robotics.
Impacts on Business Operations
Boosting Productivity and Efficiency
Organizations integrating agentic AI into their operations report significant gains in productivity and efficiency. By automating routine tasks and providing actionable insights, businesses can optimize workflows and reduce operational costs. For instance, in supply chain management, AI systems analyze vast amounts of data to forecast demand, manage inventory, and streamline logistics.
Transforming Customer Interaction
Agentic AI is reshaping the landscape of customer service through chatbots and virtual agents capable of providing 24/7 assistance. These systems not only resolve customer inquiries but also learn from interactions to enhance their responses over time, leading to a more personalized customer experience.
Case Studies: Success Stories
Numerous companies successfully implemented agentic AI solutions. For instance:
- Amazon: Utilizes AI for inventory management and logistics, significantly enhancing delivery efficiency and customer satisfaction.
- Salesforce: Implements AI-driven analytics to glean insights from customer data, enabling businesses to tailor their marketing strategies effectively.
Challenges and Risks
Ethical Considerations
Despite the promise of agentic AI, its deployment raises several ethical concerns. Issues related to transparency, accountability, and fairness are paramount. Companies must navigate these challenges to build trust with end-users. Establishing guidelines for ethical AI use, including fairness in decision-making and transparent algorithms, is essential.
Reliability and Oversight
Reliability remains a significant concern. Autonomous systems operate in unpredictable environments where errors can have serious consequences. Integration of oversight mechanisms, such as human-in-the-loop designs, can mitigate risks associated with autonomous decision-making.
Security Issues with Autonomous Systems
As AI systems become increasingly autonomous, they also present new security challenges. Ensuring that proprietary data and AI models are secure from unauthorized access is crucial. Implementing robust cybersecurity protocols and regularly updating systems are essential practices to safeguard against vulnerabilities.
Future of Agentic Human AI
Trends Shaping the Industry
Several trends are emerging that will shape the future of agentic AI:
- Increased Personalization: As agents become more adept at understanding user preferences, businesses will leverage AI to provide highly personalized experiences.
- Advancements in Explainability: There will be a push for AI systems that can explain their reasoning processes to users, fostering trust and understanding.
Potential Developments in Technology
The evolution of technologies that enable agentic AI will continue to unfold. Enhanced algorithms, improved computing power, and rich datasets will fuel innovations that drive deeper autonomy and contextual understanding.
Preparing for Change: Best Practices
To successfully integrate agentic AI, organizations should adopt best practices such as:
- Investing in Training: Ensuring employees are well-equipped to interact with AI systems and understand their benefits and limitations.
- Creating Cross-Functional Teams: Collaboration across departments to leverage AI capabilities effectively and address potential impacts on workforce dynamics.
- Establishing Clear Guidelines: Developing robust frameworks that govern the ethical use of AI systems within the organization.